QRNN
Implementation of a Quantile Regression Neural Network
Mission
Uncertainty estimation via neural networks: recovery of atmospheric 𝐶𝑂2 and associated uncertainties
Instruments
IASI, AMSU
Skills
Radiometry, algorithmic development
Date
From 2019 to 2021
The QRNN project
The extraction and analysis of geophysical parameters from remote sensing measurements plays a crucial role in the knowledge of the Earth’s physical phenomena. Indeed, greenhouse gases are responsible for important effects on our atmosphere, such as the Earth’s climate change.
In this context, methods based on machine learning algorithms have become important in the scientific community. Multi-layer perceptual neural networks (MLPs) have proven to provide good estimates of atmospheric parameters. Also, they have proven to be more efficient (in terms of computational cost and processing of non-linear systems/models) than classical inversion methods, such as the optimal estimation method (OEM).
However, classical NR techniques do not provide information on the uncertainty of the recovered parameters.
Yet this uncertainty information is essential for the exploitation of scientific products. For example, it is important for their use in systems for analysing and predicting atmospheric composition and/or dynamics.
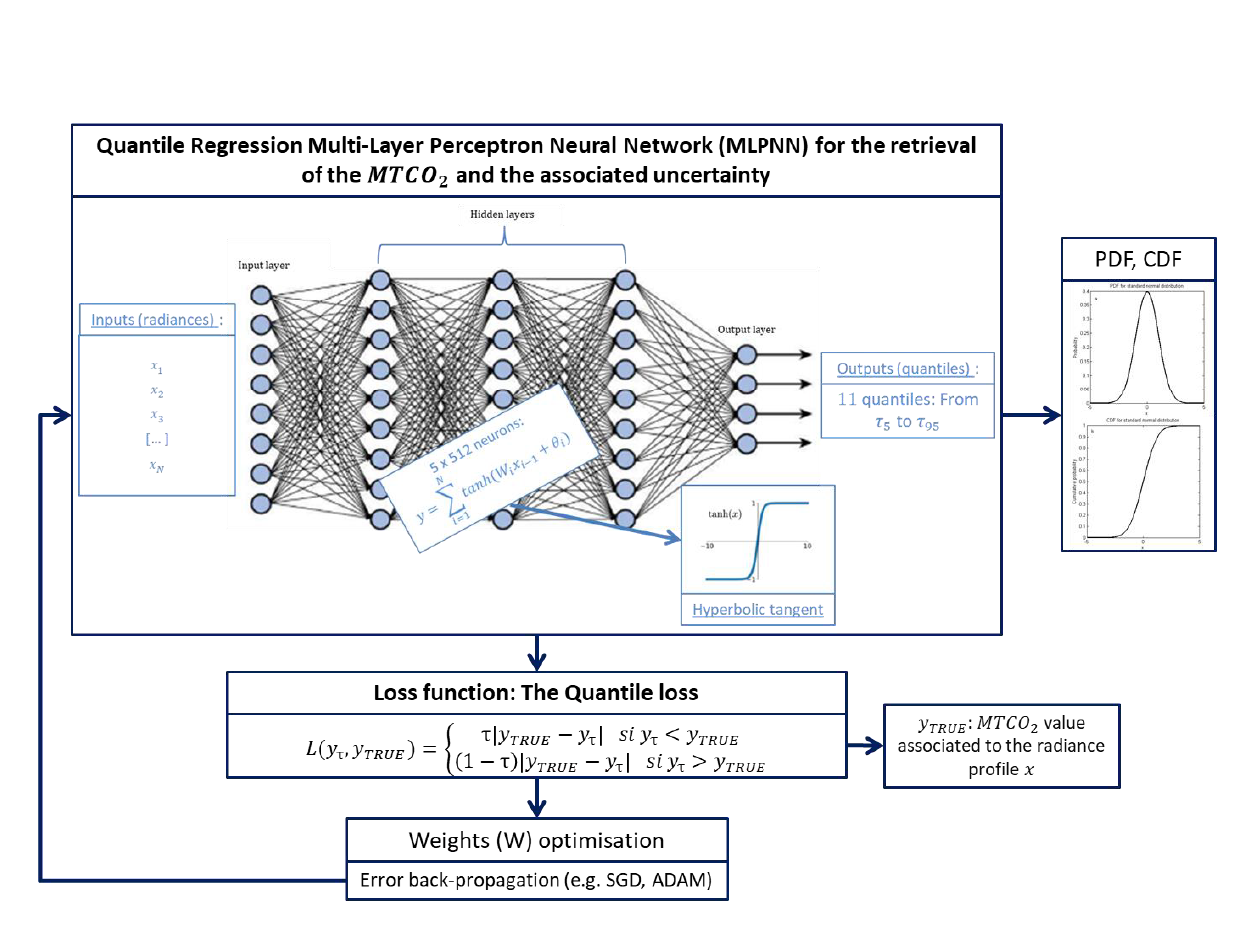
Within this framework, the unit is in charge of understanding and estimating the potential of obtaining data on the composition of the thermal atmosphere, more precisely, on the content of 𝑪𝑶𝟐 in the troposphere. These measurements are made from infrared hyperspectral survey instruments such as IASI, IASI-NG or OCO-2. Therefore, the unit must be able to determine the uncertainty associated with them, using methods based on neural networks, in order to prepare future missions (e.g. Microcarb).
This work was conducted as part of a CNES project.
The objectives of QRNN
-
-
- Implementation of a quantile regression MLP (QRNN) for the estimation of atmospheric 𝑪𝑂2 content
-
- Validation of the uncertainty associated with the inverted 𝑪𝑂2 values provided by the QRNN
-
- Comparison of the inversions and associated uncertainties provided by the QRNN with those of more classical methods (OEM, Monte-Carlo Markov Chain – MCMC)
-
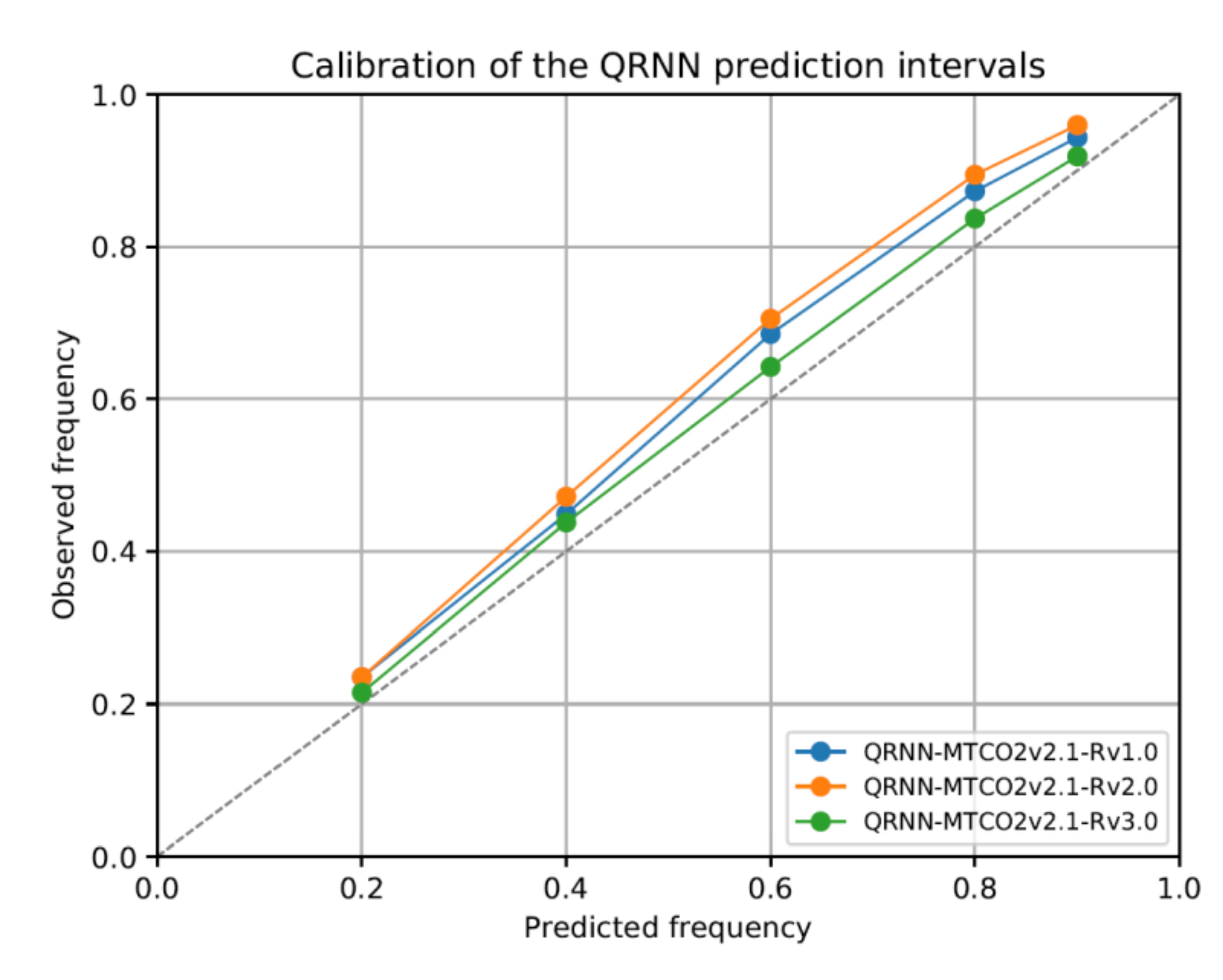
Key partners
CNES, SPASCIA, L’Observatoire de Paris, Laboratoire d’Etude du Rayonnement et de la Matière en Astrophysique (LERMA)
Key words
observation, satellite, earth, studies, uncertainty, atmospheric data, CO2, regression, quantile, neural networks, GHG, greenhouse gases, climate change, climate
SCIENCE FOR EARTH CARE
The Earth Observation Unit of Magellium is an expert in optical space missions and geophysical and biophysical applications. The EO unit provides high level of expertise and full capacity on the whole processing chain, enabling it to respond to all projects from the greatest space orders such as ESA and CNES.